How do we translate information into insights?
If you were standing in the corner of a party and someone said to you, “There are 187 people in this room,” you probably wouldn’t react - maybe you’d raise an eyebrow if anything.
Now imagine someone said to you, “There are 187 people in this room, but the maximum occupancy is 90. This building has a yellow notice, and there have been two earthquakes in this region over the last 30 days.”
Chances are, you’d be out of that building as fast as possible.
Information is different than insight
Disparate pieces of information might be interesting, but they are rarely actionable on their own - insights, on the other hand, provide us with enough context to be able to do something off the back of them. This is relevant for escaping earthquake-unsafe buildings, and it’s relevant for solving business challenges, too.
Datamine is often approached by clients who want a better way of translating their data into information, and information into insight. They’re frustrated by the fact that they’re being handed a bunch of numbers by their analysis team - numbers that are ‘supposedly’ useful, but that are incredibly difficult for non-technical people to make business decisions based off of.
In my experience, there is quite a bit of upfront work that needs to be done before you even touch the data that will enable you to embark on analytics initiatives and get valuable, actionable insights out the other end. Here is that full process fleshed out.
1. Define the problem statement
It’s important to get good at defining the problem you’re trying to solve with analytics. By first creating a simple problem statement, you can clearly articulate what you’re trying to achieve through the project. Click here to read a bit more about what goes into this.
2. Outline your hypotheses
Before you begin any analysis, you need to come up with a whole bunch of hypotheses about what you think you know. What is your gut telling you? These will often form part of the framework within which the analysis secures relevance for the business, rather than a broad “fact finding mission” that may ultimately miss the mark.
What do you want to be able to do after this project? What stucks are you hoping to get unstuck through the analysis? Make sure this is properly articulated at the outset so you can refer back post-analysis and determine whether or not the project was successful.
4. Determine the analysis goal
What do you want to discover through the analysis, and how does it relate to both your overarching hypotheses and the business goal? This is also a frequently missed (but very important) step of the process.
5. Think about implementation
You might get to the end of a project and have some great insights that you want to action, but if you’re unable to implement them, you’ll just end up right back at square one (only poorer and more frustrated). Are you in control of the value capture? What business action will these answers enable you to take, and are there any roadblocks you need to clear first?
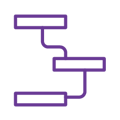
The next step is figuring out exactly what information will need to go into your analysis in order to achieve all of the above goals. What do you want to discover, and therefore what do you need to analyse? Map out all of the various data sources you might require, as well as what other factors should be taken into consideration throughout the analysis.
Give your data analysts the framework you’ve just created and send them off to produce outputs that align with those requirements. Contrary to popular belief, this is actually one of the quickest stages of the process.
8. Distil the outputs into insights
Now for the fun bit. In every organisation, there needs to be a translation layer - a person that is responsible for making a connection between the analysis and the business outcome. Someone who is a part of the analytics process but isn’t necessarily an analyst. The analyst’s job is to create a large body of work with many outputs, but someone else needs to sift through all of those outputs (ruthlessly, at times), determine which are the most valuable and craft the story around them to then present back to the business. If you really want to get value from your analysis, this is an incredibly important role to fill.
At Datamine, we have an entire team dedicated to data storytelling and translation - they’re known as our Owls. We understand that our clients are busy and have limited time to spend understanding the analysis we’ve done, so in every project our Owl team pulls out the 1-3 key insights they’ve distilled and presents these (and only these) back to the client.
Even though it might seem counter-intuitive to do hours of analysis and produce a wealth of outputs only to boil them down to a few key points or actions, we’ve found this approach to be incredibly effective. Research has shown that on average people only remember four out of 20 presentation slides after the fact and that they’re more likely to remember the first section rather than the last. So rather than overwhelming our clients with dozens of graphs, pie charts and disparate facts, we begin our results presentations with the key insights we want them to remember, and we then focus on explaining those throughout.
Regardless of the size and nature of your business, this is a useful structure to follow when undertaking analytics. Make sure you have a dedicated resource who can sift through analytical outputs to find valuable pieces of information, then add the context that will turn that information into insight. If you’re not sure where to begin, we’d be happy to chat with you - just click here to schedule a free phone consultation with us.
ABOUT THE AUTHOR: MATT WILKINS (PARTNER)
Matt is a passionate advocate for using data-driven intelligence to identify and address business challenges. A big supporter of implementing analytics in Marketing, Matt has the expertise to balance the technical, commercial and cultural considerations required to derive value from analytics.