Why your analytics initiatives aren't delivering you value
We all know how it feels to be excited about the potential of something, only to have it turn out less awesome than anticipated. Unfortunately, this is a common problem when organisations jump into analytics, and the people who were initially the most excited about the prospect often end up being the ones who feel most responsible when it doesn’t deliver value.
In the midst of the current hype and momentum around data analytics, there is a gap between that momentum and the corresponding value output (or lack thereof). Here are the four primary reasons I believe this happens, along with Datamine's suggestions for how to realise the value of analytics and scale efficiently while avoiding heartbreak and hassle:
1. There’s a disconnect between the business and technical areas
In a given organisation, there will be a business side of things and a technical side of things. Often these two business units are not as in tune with one another as they need to be in order to get value from analytics. It’s important for there to be accountability and communication between the two entities, as described below:
- Business: the organisation needs to be able to define the problem they’re trying to solve - and this needs to happen well before the tech teams get involved. It might seem elementary, but establishing an agreed problem statement at the beginning can save you from massive headaches down the line. The business side also needs to think all the way through the value chain of that specific analytics initiative, and to consider any policies and politics that might get in the way of unlocking that value.
- Technical: the teams need to be able to take the clear problem statement and design a practical solution - one that isn’t overengineered, or a $1M solution for a $200K problem. These teams need a way to consistently deliver analytics projects and work within a defined framework that allows you to produce repeatable stuff down the line.
2. Analytics roles and responsibilities across departments are unclear
One common struggle companies face is the question of how to organise the team, or teams of data analysts. Businesses often hire techies but don’t have the structure in place to support them, which often leads to one of two things:
- The analysts get spread out across different business units, meaning they can’t learn from or communicate effectively with one another, which in turn leads to work being repeated due to lack of visibility
- They get grouped all together in one siloed team, meaning they lose track of what’s happening in the business and the issues facing the various teams they should be supporting
The ideal setup for driving acceleration is actually a combination of both - where some analysts are spread out amongst multiple business units, and others work within what we refer to as a ‘centre of excellence’. The technical team within the centre of excellence is responsible for building capability and keeping the pipeline visible and well-communicated. To get work done, there’s a translation layer between the analysts in the centre of excellence and those in the business units. This type of organisational setup drives capability building and acceleration.
3. Projects are being completed, but the benefits aren’t being realised
The third major issue we often see preventing businesses from truly getting value from analytics has to do with setting up a framework in which it’s easy to realise benefits. One component of this is to make sure you’re first focusing on quick, repeatable projects that are easy to measure - being able to prove these projects work will give you the momentum and buy-in from management to continue with tougher challenges. It’s also important to have a prioritised pipeline of all analytics work that is visible both within the centre of excellence and across the business verticals. Without this, you’ll have different pipelines that are working independently rather than together, making it very difficult to tie value back to.
From there, you need to make sure you have the right technical setup, one in which you have three distinct areas:
- An exploratory area, or ‘sandpit area’ where you can test things quickly to prove that they will be beneficial and profitable before actually launching in at full scale
- A prototyping area where you can create a solution design and validate it
- A scaling area where you can take what you’ve made and roll it out
Many businesses only have one area in which they do analytics and one ‘speed’ at which they try to tackle all these steps, which means they end up jumping too quickly from step to another and don’t get what they want out the other end. Separating your work streams into these distinct buckets can help you unlock the value at the end of the project.
4. Data is stored in the wrong platform and isn’t easily accessible
Most businesses have heard talk of data warehouses, data lakes and datamarts, but we’re seeing a lot of confusion around the different types of repositories and what they’re best used for. Here’s a quick rundown:
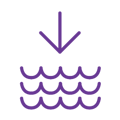
- A data lake is effectively a data dump – it contains raw, unfiltered data that’s easy to put in but difficult to get out
- A data warehouse is designed for operational and external reporting - it drives the BI function and contains an organisation’s clean and structured data. It’s easier to get information out of a warehouse because the structures and definitions are locked down when it’s built. However, this often means the data is not structured correctly or at the right level of aggregation to actually be useful for analytics
- A datamart is a smaller repository with data that is unique to a specific business function. For example, a datamart dedicated to customer insights might contain customer and behavioural information that’s easily accessible, at a useful level of granularity and in a format that doesn’t require the linking of multiple tables
Businesses often unknowingly apply data warehousing principles to data lakes, or to datamarts, meaning they waste resource and hundreds of people hours trying to get value from the wrong kind of repository for the task they’re trying to do. Our suggestion is first to consider what you’re trying to achieve, then creating a datamart that serves that unique purpose - then you can pull data from the warehouse and lake (but only the data you need) and store it in the datamart. This avoids big headaches down the road, and the ultimate cost savings can be in the millions.
Read this only if you’re looking to accelerate.
Chances are if you’re already trying and failing to get value from analytics, you’re probably making an effort to grow as a business rather than stay at the same level you’re currently at.
If that’s you, good news: the four areas described above aren’t designed for stability, they’re designed for acceleration and analytical growth. In order to stay competitive, businesses need to build and scale their analytics capability, and it can be challenging to do this alone – but people are often scared they’ll become hostages to a third-party supplier that holds and controls their data.
Rather than hold the power and do everything for you, Datamine is about helping you accelerate and build your internal capability through close collaboration with our diverse team of consultants, data scientists, visualisation experts and developers. Check out our case studies to read how we’ve helped companies in your industry get value from their analytics initiatives and regain competitive advantage.
ABOUT THE AUTHOR: MATT WILKINS (PARTNER)
Matt is a passionate advocate for using data-driven intelligence to identify and address business challenges. A big supporter of implementing analytics in Marketing, Matt has the expertise to balance the technical, commercial and cultural considerations required to derive value from analytics.